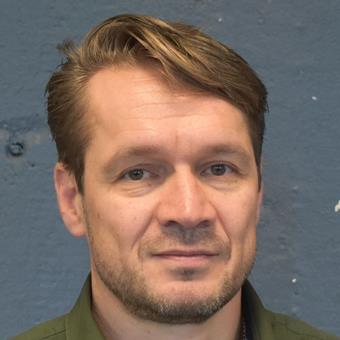
Keynote Talk
Title: Computer Vision – Machine Learning Meets Applied Mathematics
Presenter:
Prof. Michael Felsberg
Computer Vision Laboratory
Linköping University
Linköping, Sweden
Abstract:
The field of computer vision is a sub area of AI, and it has its roots in the modeling of the human visual system (HVS). It is commonly accepted that about 80% of what we perceive is vision-based (Ripley and Politzer 2010), but modeling vision is a systematically underestimated scientific challenge – an implication of Moravec’s paradox, “we’re least aware of what our minds do best” (Minsky 1986). The highly intuitive nature of the HVS makes it difficult for us to understand the myriad of interdisciplinary problems associated with computer vision. The research at the Computer Vision Laboratory (CVL) has a strong focus on theory and methods, in particular within machine learning, signal processing, and applied mathematics. The resulting methods are applied in fields where technical systems are supposed to coexist with and therefore predict actions of humans. Self-driving cars sharing road space and interacting with humans, sustainable forestry and agriculture, monitoring of greenhouse gases as well as classification and monitoring of animals are some application domains.
My talk will cover a wide range of topics within machine learning for computer vision and robot perception: Few-shot and weakly supervised learning, geometric deep learning, semi-supervised and incremental learning, scene flow estimation, uncertainty representation, as well as video and semantic segmentation.
Bio:
Michael Felsberg received the PhD degree from Kiel University, Germany, in 2002, and the docent degree from Linköping University, in 2005. He is a full professor with Linköping University, Sweden.
His research interests include, besides visual object tracking, video object and instance segmentation, classification, segmentation, and registration of point clouds, as well as efficient machine learning techniques for incremental, few-shot, and long-tailed settings.